A Beginner's Guide to Fine-Tuning Large Language Models
Why do we need to finetune LLMs and an overview of popular finetuning techniques
Welcome to the exciting world of LLMs! If you've been following our journey, you'll know that we previously ventured into the realm of Large Language Models (LLMs), exploring their capabilities and potential applications. Today, we're diving a level deeper and turning our attention to a crucial aspect of LLM model development: fine-tuning these LLMs. This guide is beginner-friendly, requiring no prior LLM expertise, and will help you understand the fine-tuning process in a simplified and engaging manner. So, let's begin our journey into the world of LLM fine-tuning.
Understanding Fine-Tuning in Large Language Models
The concept of fine-tuning in LLM is not as complex as it may initially sound. Let's consider a practical scenario to clarify it. Suppose you are an accomplished linguist, capable of speaking several languages fluently. Now, you want to become a tour guide in Paris. While your overall language skills are impressive, you'll need specialized knowledge about French history, culture, and local slang to truly shine in your new role. This is where specialized training or 'fine-tuning' comes into play.
In the context of LLMs, 'fine-tuning' serves a similar purpose. Despite the impressive ability of LLMs to generate text in various contexts, they require fine-tuning to perform specific tasks or understand particular domains more accurately. Fine-tuning trains the LLM on task-specific or domain-specific data, thereby enhancing its performance in those areas.
Exploring the Significance of Fine-Tuning
Why can't we just use the base model? Why go through the process of fine-tuning? This question may linger in your mind, but the answer is quite straightforward. Just as a linguist cannot rely on generic language skills to explain intricate details about French culture, an unrefined LLM may falter when faced with tasks requiring a certain level of domain expertise.
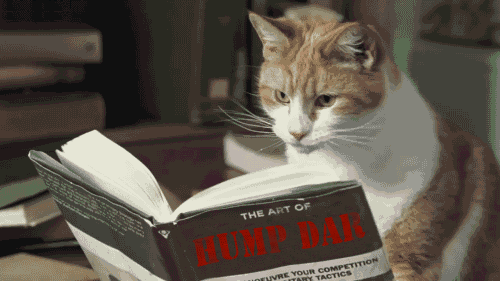
For example, consider an LLM used for diagnosing diseases based on medical transcripts. This LLM, fine-tuned with medical data, will offer far superior performance compared to the base model, which lacks the required medical knowledge. Therefore, fine-tuning becomes indispensable when dealing with specialized fields, sensitive data, or unique information that isn't well-represented in the general training data.
When to Opt for Fine-Tuning
The decision to fine-tune an LLM hinges on several factors, including your specific use case, the associated costs, and the desired level of domain specificity.
For general tasks such as answering questions or summarizing documents, pre-trained models like ChatGPT, which are readily available via APIs, yield satisfactory results. Moreover, leveraging these APIs is a cost-effective solution, making them an attractive option for many.
However, for tasks involving heavy data processing or requiring a specific level of expertise, fine-tuning is the way to go. It empowers the model to comprehend and generate text in alignment with a specific field's expert knowledge, thereby enhancing the quality of outputs significantly.
Unpacking the Methods of Fine-Tuning
Fine-tuning an LLM can be carried out in a variety of ways, depending on the task at hand and the resources at your disposal. At times, the base model is used as a 'fixed feature extractor,' wherein the model processes new input data and generates high-dimensional vector representations. These features are then utilized to train a new model that is task-specific. Try fine-tuning an LLM using OpenAI in the colab notebook.
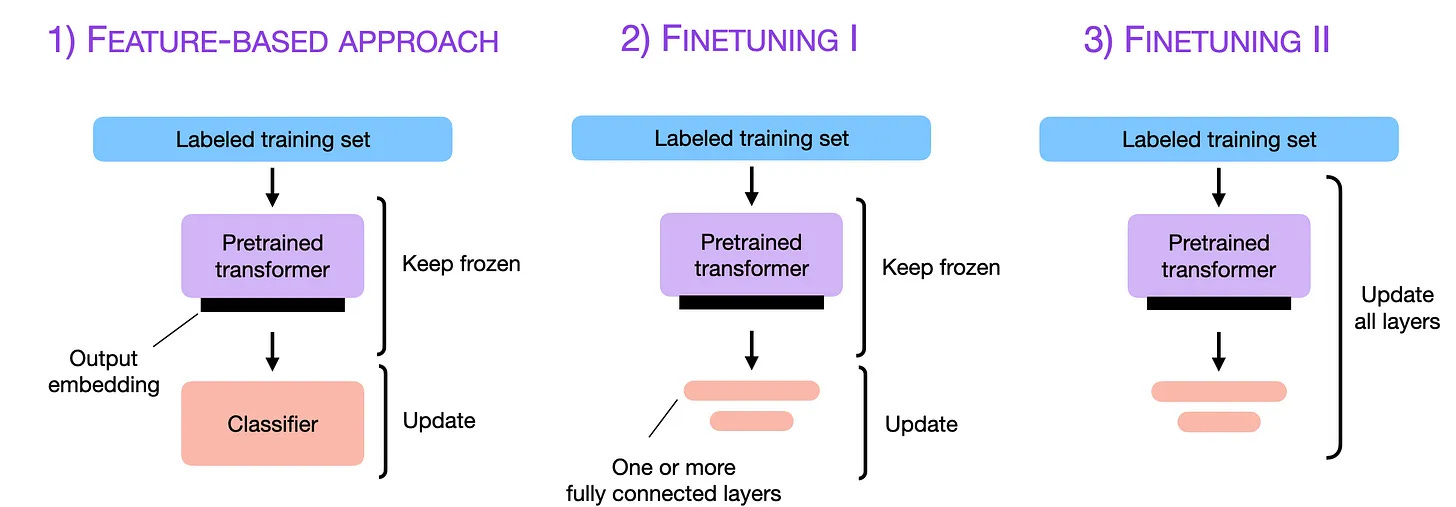
In some cases, the output layers of the base model are modified, leaving the remaining model unchanged. And in the most resource-intensive scenarios, the entire model—from input to output—is subjected to fine-tuning.
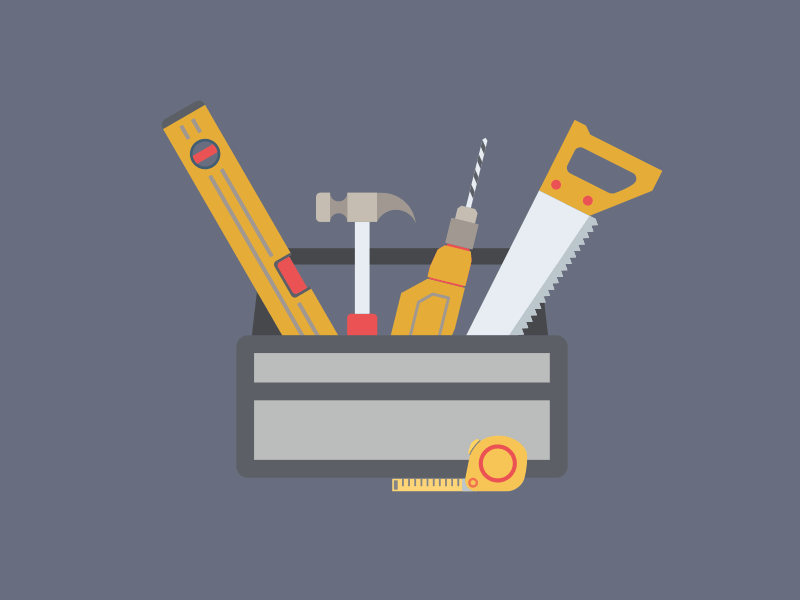
Recently, 'parameter-efficient fine-tuning' methods like Adapter Tuning have gained traction in the field. This approach introduces 'adapter layers,' a new set of parameters, to each transformer block of the model. This significantly enhances the efficiency of the fine-tuning process, making it a promising avenue for future LLMs applications.
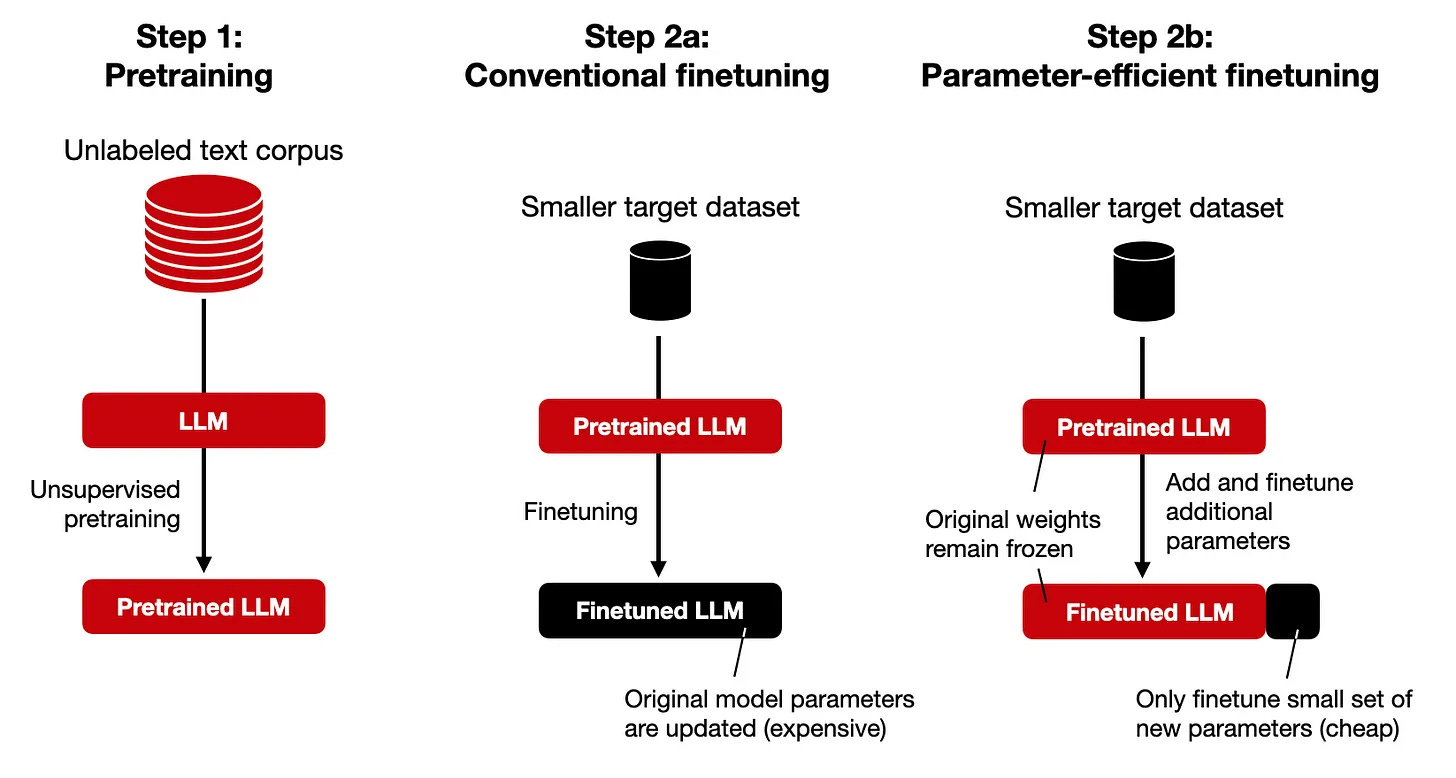
Conclusion
Fine-tuning LLMs is an exciting blend of art and science. It might seem challenging at first, but remember, even the most talented musicians need to fine-tune their instruments to produce the most harmonious melodies. With patience, practice, and a sound understanding, you too can master the art of fine-tuning in LLMs and unlock its enormous potential.
Stay tuned for more enlightening insights into the captivating world of LLMs and MLOps, and continue to fuel your curiosity with us!